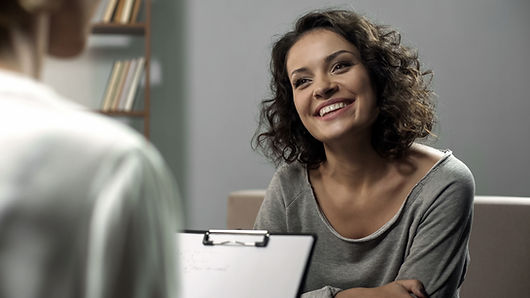
MINet: A Novel Telemedicine Tool for Providing Automatic Feedback for Motivational Interviewing (MI) Using Machine Learning
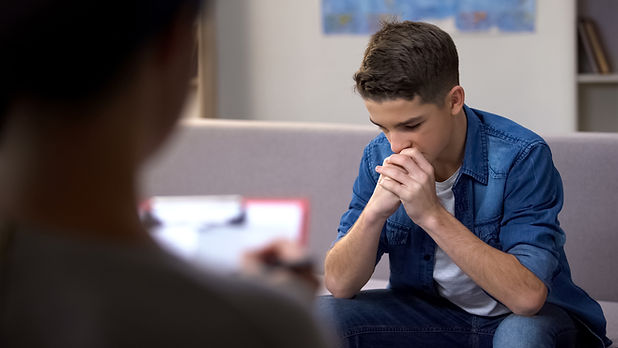
Abstract
Due to COVID-19, counselors have found it difficult to interact with their patients in an efficacious manner because of a lack of emotional connection. Furthermore, the stress imposed by the pandemic has caused levels of mental illness to increase three-fold.
Motivational interviewing (MI), a counseling method that encourages patients to make positive changes to their behaviors and lifestyle. Originally developed for addiction, its use is now expanding to depression and anxiety and criminal justice. However, no objective, comprehensive system has been developed to track the quality of MI conversations and provide feedback for low-quality conversations. To solve this problem, natural language processing (NLP) was used to track the quality of these conversations and derive novel insights into linguistic features that constitute high-quality MI conversations. A dataset with 258 high- and low-quality MI conversations from the University of Michigan was used to train the algorithm. NLP techniques such as Jaccard similarity, latent dirichlet analysis, and sentiment analysis were used to analyze the data for common linguistic patterns in MI conversations. Finally, the output of the calculated features was used as input into a k-means cluster (k=3) algorithm to allow for the automatic classification of a MI session as high- or low-quality, with an accuracy of 91.11% and an F1-score of 91.67. The generated system accelerates the advancement of high-quality MI conversations, helping counselors that lack the emotional connection to their patients in virtual circumstances, along with providing easily applicable insights into how counselors can improve their practice.
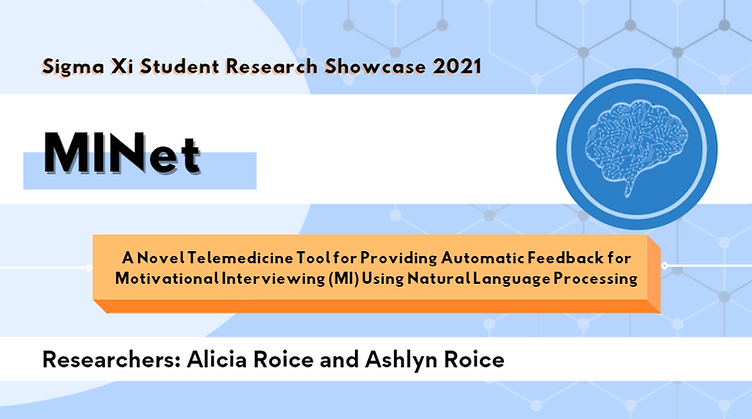